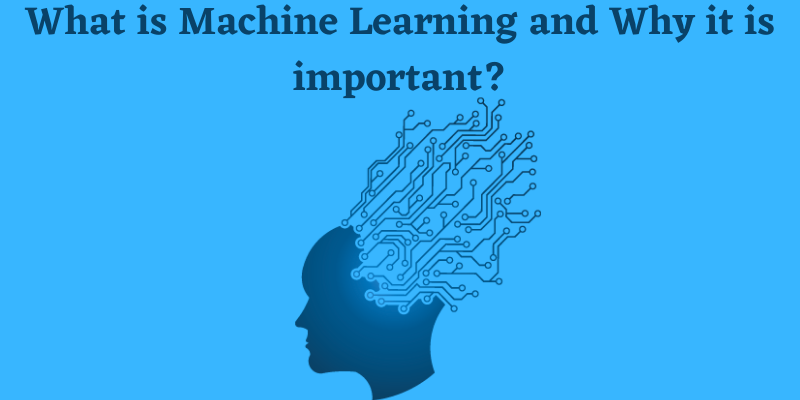
What is Machine Learning?
Machine learning (ML) is a sort of artificial intelligence (AI) that allows software applications to improve their prediction accuracy without being expressly designed to do so. In order to forecast new output values, machine learning algorithms use historical data as input. Join Machine Learning Course in Chennai for the best technical knowledge.
Recommendation engines commonly employ machine learning. Typical applications include fraud detection, business process automation (BPA), malware threat detection, spam filtering, and predictive maintenance. Here, In this blog we are going to discuss about “What is Machine Learning and Why it is important?”
Why is Machine Learning Important?
Machine learning is important because it allows companies to discover patterns in customer behavior and corporate operations while also assisting in the development of new products. Many of today’s most successful organizations, such as Facebook, Google, and Uber, use machine learning. Machine learning has become a critical competitive differentiator for many firms. Machine Learning Online Course will help you to enhance your technical skills in Machine Learning.
Types of Machine Learning:
The way an algorithm learns to become more accurate in its predictions is how traditional machine learning is often classified. Supervised learning, unsupervised learning, semi-supervised learning, and reinforcement learning are the four primary methodologies. The type of data that data scientists want to predict determines the algorithm they apply.
Supervised learning: In supervised learning, data scientists input labelled training data to algorithms and select the variables they want the machine to look for correlations on. Both the input and output of the algorithm are supplied.
Unsupervised learning: Unsupervised learning is a term used to describe machine learning algorithms that train on unlabeled data. The programme looks for links between data sets that are important. All of the data used to train algorithms, as well as the forecasts or recommendations they generate, is predefined.
Semi-supervised learning: Semi-supervised learning is a hybrid of the two previous approaches to machine learning. Although data scientists may feed an algorithm largely labelled training data, the model is allowed to explore the data and establish its own understanding of the set.
Reinforcement learning: Data scientists use reinforcement learning to teach a machine how to complete a multi-step procedure with clearly given rules. Data scientists create an algorithm to complete a task and deliver positive or negative feedback to it as it learns how to do so. However, for the most part, the algorithm chooses which steps to take along the way on its own.